Nobody can tell the future. Six months ago, I would have never guessed (even for April Fool’s Day) that we would find ourselves here, in a real-life version of the film Contagion. Modelling a black swan event like COVID-19 with traditional quantitative techniques is extremely difficult, due precisely to any black swan event’s inherent unpredictability. However, several machine learning methodologies exist that, when used in conjunction with our machine learning software Boosted Insights, can help model the pandemic risk factor. We can hedge risk, bet on a fast recovery or bet on long drawn out damage depending on our view.
To model these scenarios, first we identify the companies most directly related to the pandemic. To do this, we will delve into a field outside of traditional quantitative techniques. Natural Language Processing (NLP) is a subfield of linguistics that utilizes machine learning to analyze large amounts of language data. Within NLP there is a branch called topic modelling. Topic modelling is a set of techniques that scan a set of documents (called a corpus), detecting word and phrase patterns within them and automatically clustering word groups and similar expressions that best characterize the corpus. In this case, we can use it to identify words and topics related to COVID-19. Then, we can find words and topics related to different companies and find the ones that connect most strongly to the COVID-19 topics. In topic modelling, you must first find the set of data you want to learn from. You want a set of data that captures all the information pertinent to the topics you’re trying to model. News articles, encyclopedias, blog posts and analyst reports can all be useful sources. In this case we want to model COVID-19, so we’ll need data from December 2019 (when news of the Coronavirus first started appearing) until present to best capture everything known about it. Wikipedia is a particularly valuable corpus because it has information on many topics, and many articles link directly to other articles directly, providing a hint on what entities are related to other entities. Wikipedia, though community edited (and therefore a possible source of bad information), has robust sourcing from a variety of different news outlets and information is aggregated constantly across the world, which provides good data for the machine to work off of. After identifying the source of the data there are several techniques that can be employed. The simplest is co-occurrence. Co-occurrence assumes that if two words appear in the same documents often, they must be connected and conversely, if two words don’t appear together frequently then they must not be connected. From there we can move onto Latent Semantic Analysis (LSA) and its successor Latent Dirichlet Allocation (LDA) which extends the idea of co-occurrence to model the fact that documents contain a collection of topics and all words belong to a topic. If Word A is related to Word B and Word B is related to Word C then A is related to C. LDA is still heavily in use but newer techniques use neural networks. The two most common neural network models are Word2Vec and Deep Semantic Similarity Model (DSSM). Depending on the variant of these techniques the full context of every word is used (the articles it appears in, the words around it, any articles it connects to, etc.) to predict other related words or topics.
Now we can figure out which words and topics most heavily relate to COVID-19. The next step is identifying words and topics related to each company. Depending on the data used for topic modeling we could just use our topic modelling system to grab topics for each company. However, we may want different data for finding company keywords. As an example, Wikipedia could potentially be a good source of data to find topics related to COVID-19, but we might also want to use 10-Qs, analyst reports, and earnings call transcripts to find keywords related to the company. Topic modelling requires millions of documents so even with those examples, we don’t have enough data. Instead, we turn to another area of NLP called Named Entity Recognition (NER). NER is a set of techniques that locate and classify named entities (such as keywords, commodities, locations, risk factors, etc.). The simplest and easiest are variants of Term Frequency-Inverse Document Frequency (TF-IDF). TF-IDF finds words that are common in the current document but rare in every other document. From there you can use learning techniques like Conditional Random Fields (CRF) and Long Short Term Memory neural networks (LSTM) to explicitly learn what words are important based on sentence structure, grammar, and other language clues.
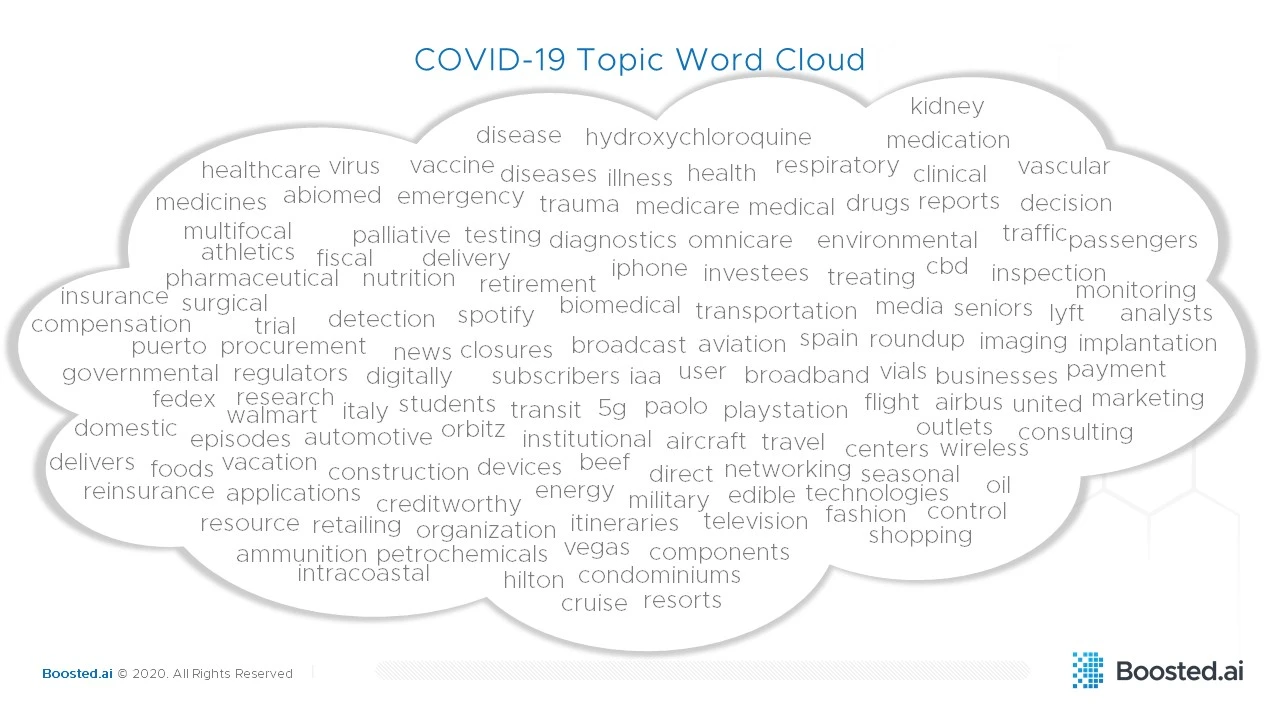
Figure 1 Words near the top of this cloud have a stronger COVID-19 connection
We used the Russell 1000 as a stock universe to find specific equities within these industries that are connected to these topics. Boosted Insights distinguished REITs like Simon Property Group (SPG) and National Retail Properties Inc. (NNN), which it found to be very related to COVID-19, from commercial real estate firms like CBRE Group (CBRE), which it didn’t find to be highly related.
It doesn’t take machine learning (or rocket science, even!) to figure out that all cruise line stocks in the Russell – Carnival (CCL), Royal Caribbean (RCL) and Norwegian Cruise Line (NCLH) – are all considered highly related to COVID-19. A user may derive the most value from Boosted Insights through crowded sectors like retail. It’s assumed that within an economic downturn, retail stocks will suffer. However, there are many different retail equities in the Russell 1000. This model found that certain stocks like Nordstrom (JWN) and Ralph Lauren Corporation (RL) were less likely to be affected by COVID-19 than Under Armour (UA, UAA), Columbia Sportswear (COLM) and Capri Holdings (CPRI). These findings may help an investment manager make portfolio decisions on their retail holdings.
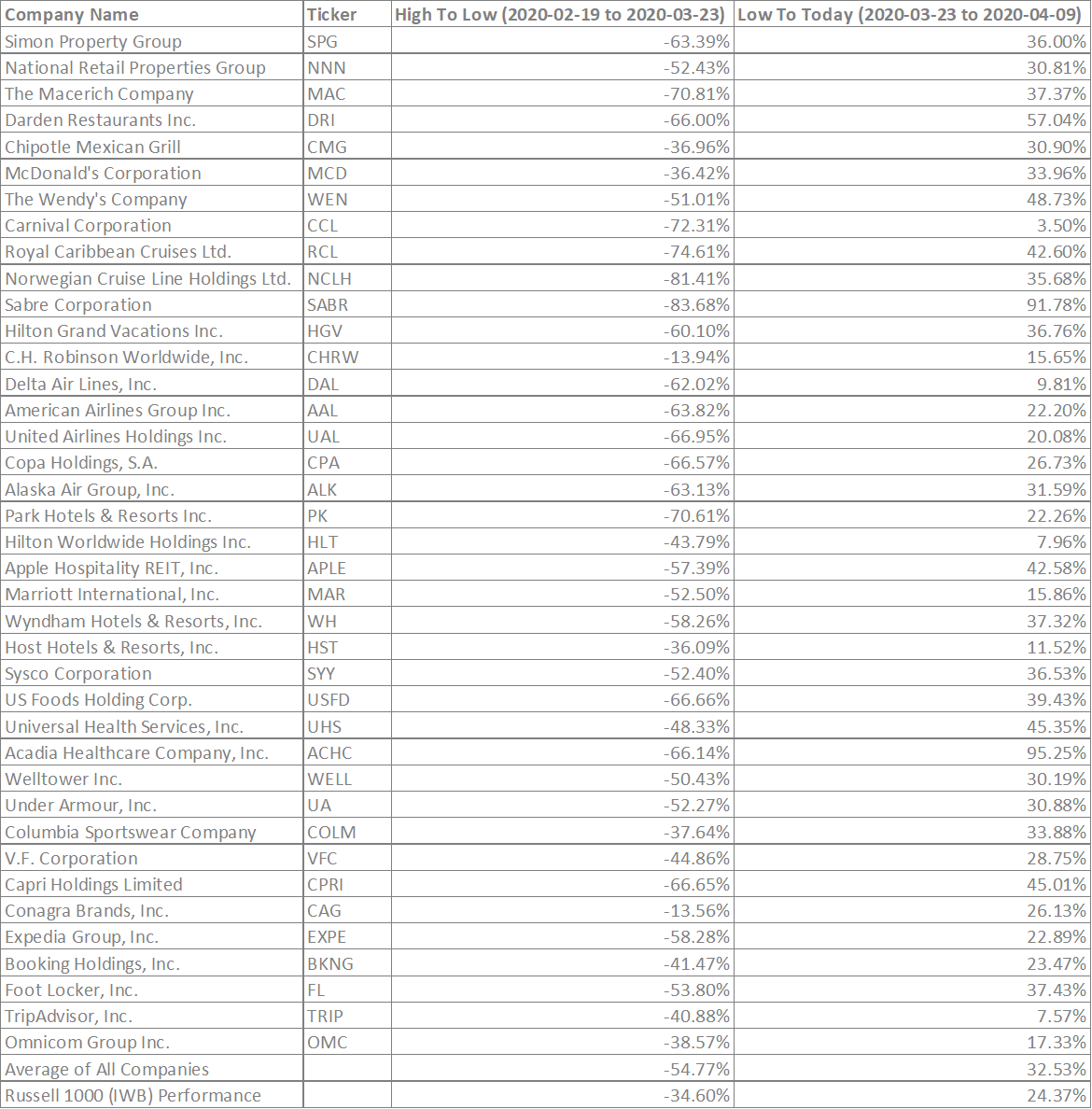
Figure 2 These Russell 1000 companies fell more and recovered more than the index (IWB)
To find whether a company’s relationship to COVID-19 is positive or negative, we turn to more traditional quantitative techniques. Risk factor analysis is a quantitative attempt to model the exposure of a portfolio to different characteristics that can explain risk and return. Traditionally, the most popular is the Barra risk model that uses about 40 hand picked factors to predict a stock or portfolio’s risk relative to the market. Examples of factors include volatility, earnings growth, senior debt rating. However, there are many more unidentified sources of risk and a lot of quantitative analysis is involved in trying to find these unknown or latent factors. One technique uses Principal Component Analysis (PCA). You start by making a matrix with the covariance of the returns for every company in your universe to every other company in the universe. PCA then reduces the dimensions to find the factors and stock weights to those factors that represent the most variation in the data. When applying PCA frequently on a short enough time scale, some factors will stay stable, and some factors will change rapidly. The stable factors represent consistent systematic risks like general market exposure, sector exposure, volatility etc. The less stable factors that change rapidly are quickly emerging factors like a political agenda, a regime change, a pandemic, etc. or, instead, they are spurious. Determining what these latent risk factors are is traditionally a very hard problem. This is where all that topic modelling work we did using Boosted Insights becomes extremely useful. We can now go through the different factors we’ve identified and cross reference the security weights with the COVID-19 cluster we identified earlier using topic modelling. The factor that has a heavy positive or negative weight to each of those securities represents COVID-19’s direct risk. We can confirm this by taking the portfolio represented by the weights and seeing how it does relative to the market during the most aggressive COVID-19 driven moves up and down.
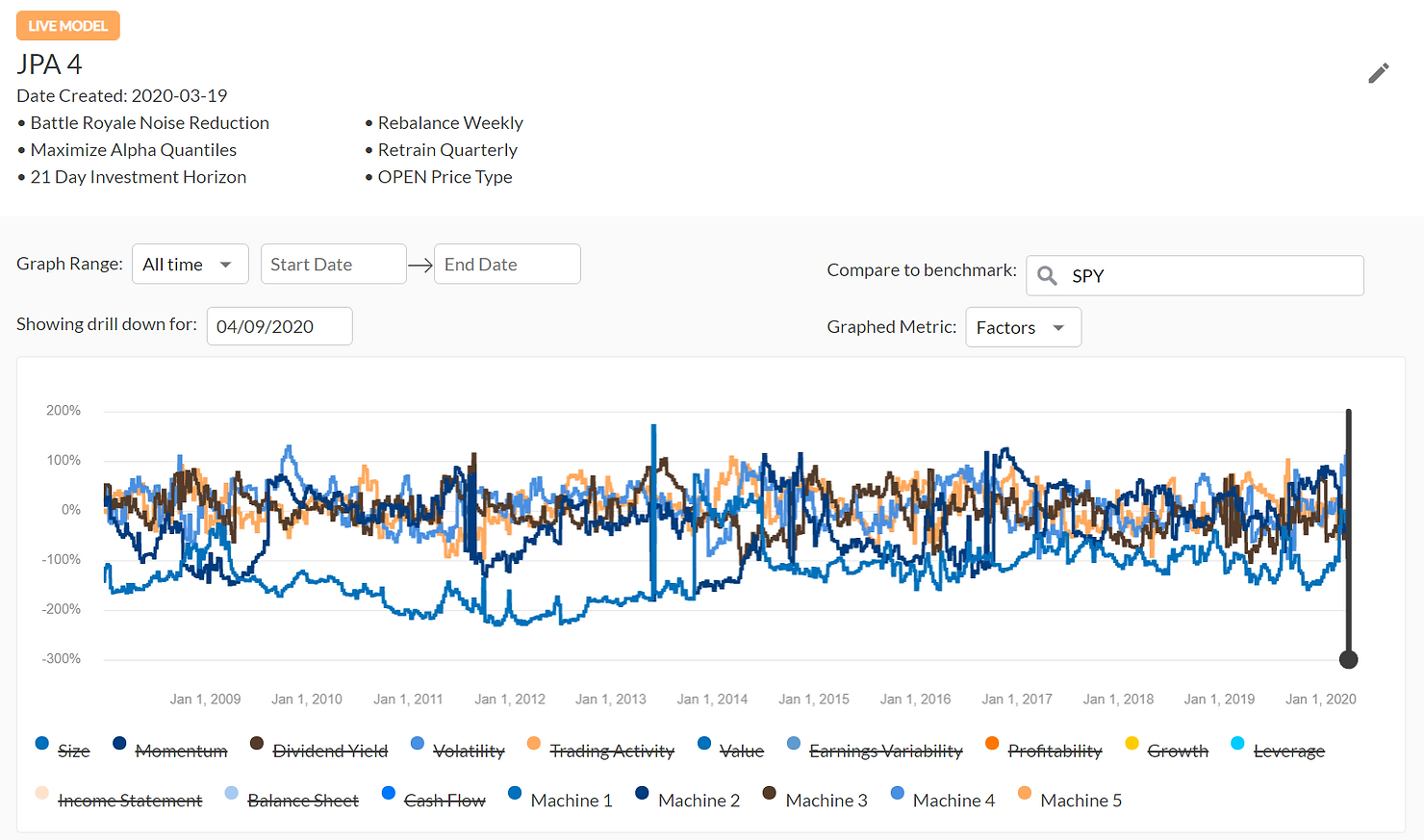
Figure 3 Machine 1 – 5 shows evolving risk factors. They change, and could be risks like oil, pandemic, interest, etc.
At this point we’ve found a cluster of equity names most heavily related to COVID-19, and we’ve identified those stock’s relationship to the pandemic. We can now proceed to neutralize our exposure to market levels, fully neutralize exposure, go long recovery or short recovery. Adjusting exposure involves portfolio construction, which we will discuss in our next post.
You can read the second article in this series here.