Artificial intelligence is at the forefront of digital transformation for investment management. We have previously discussed ways folks can use AI in their institutional investing strategies. And though there are a lot of applications of AI and machine learning, they ultimately boil down to finding a way to interpret the vast amounts of data that exists in capital markets and distilling it into actionable insights. Our stance is that the best application of AI in capital markets is quantamental in nature, meaning it is powered by the asset manager’s specific financial expertise (so requires their hand in steering it), but makes the most of the fathoms of data available to be analysed and learned from.
Many asset managers are already using big data (through studying stock reports or macroeconomic news) and performing some type of analysis on that data (following a rules-based approach to investing). What artificial intelligence seeks to do is to supercharge that evaluation through deeper and richer analysis. Here, we’ll walk through some standard strategies that investment managers use and outline why we think incorporating AI into their process will lead to the best results.
Investing based on guidelines
In a traditional, rules based approach, an investment manager may, for example, only invest in stocks with earnings growth above 10% and a P/E ratio under 20. This type of investing is easy to understand and follows intuitive investing principles, but it doesn’t adapt to changing market conditions and an insufficient number of stocks may meet the manager’s criteria.
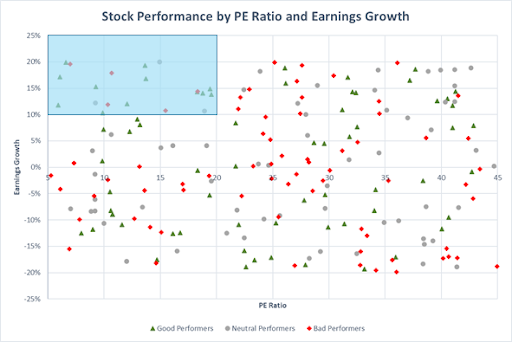
Investing based on data (linear regression)
A slightly more advanced traditional approach is the use of linear regression methods. Here, the asset manager effectively draws a line of best fit through a space, with the line attempting to fit the prediction to the data. This method is also easy to understand and is a long held industry standard. It is also computationally very inexpensive (meaning server costs and data costs tend to be low in this method). However, though it is seen as positive that it is a long held industry standard, that plus side can work against an investment manager in the changing landscape of asset management – no one wants to be doing the same thing as every one of their colleagues. Also, in linear regression methods for asset management, these models are less effective with more data, and the data that does exist tends to need to be extensively curated to work well.
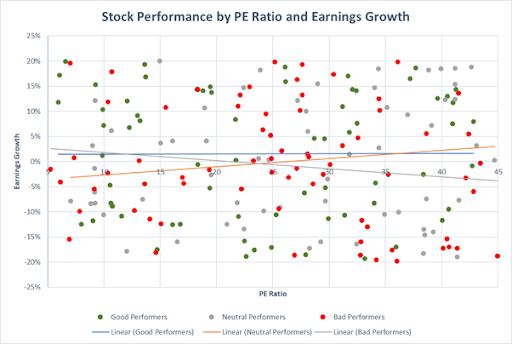
Artificial intelligence approaches
Rules based and linear regression methods are very time consuming and generally must be done manually. Asset managers should seek out a better way to add value to their portfolios. AI and machine learning methods can help managers by doing this work for them. In addition, ML methods like the ones here help asset managers find first-mover advantage in the sea of competition.
Invest with machines and data (random forest)
Random forest is an open source machine learning method that creates decision trees, training itself on historical data to find patterns within the data. This tends to offer better results than linear regression and has the benefit of still being moderately understandable. However, random forest can be vulnerable to regime shifts (something we saw recently during the quant shock) and the results tend to be more correlated with traditional techniques, as the decision trees are essentially using rules based notions in discovering data patterns. As such, random forest can struggle to find subtleties in the feature space.
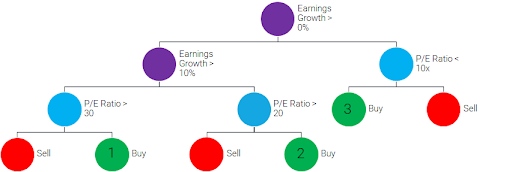
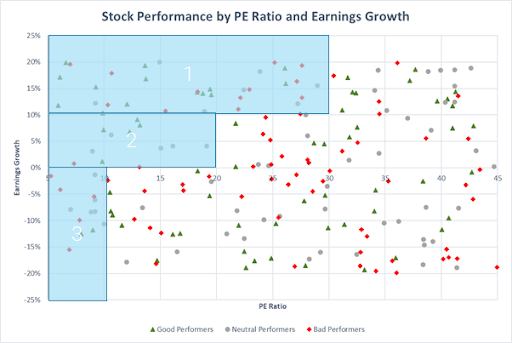
A better way: Boosted.ai’s finance specific artificial intelligence and machine learning
We built our proprietary, finance specific machine learning algorithms specifically for capital markets prediction. Our ML algorithms aim to invest with machines built like humans. It emulates the investing process a fundamental asset manager would use, comparing each pair of securities in a stock universe against each other on a per feature basis. There are many benefits to using this method for capital markets prediction. First, the results are uncorrelated to traditional techniques, so fund managers can expect their results to be unique. Second, we have invested a lot of resources in leading the charge on explainable AI and with new features like Advanced Rankings, Factor Timing and Feature Importance, a user’s portfolio is more interpretable (and explainable to stakeholders) than ever before. Because these AI portfolios are dynamically ranked and also feature our other portfolio construction improvements, they are less susceptible to volatility in their selections. They also tend to be robust to regime shifts (as they are constantly adapting and learning and can surface early risk factors). Finally, an AI portfolio built with Boosted.ai’s proprietary machine learning algorithms is excellent at finding subtle patterns in the data. The downsides to these upsides is that the approach is proprietary, so an asset manager must use Boosted.ai to reap these benefits and that performing all of these calculations can be computationally expensive compared to simpler jobs like a rules based approach.
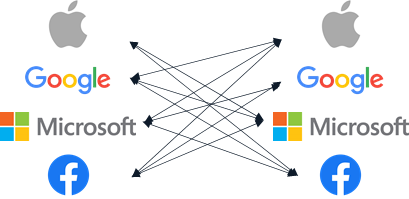
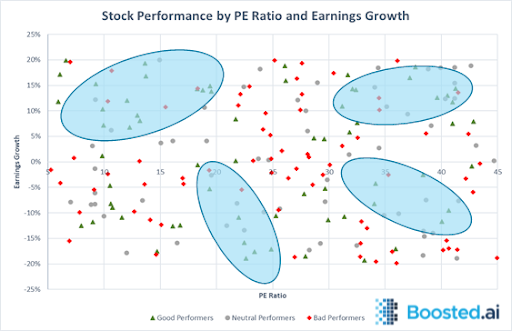
Takeaways
The environment for asset management is not getting easier. Institutional investors are in a battle to capture the most AUM and to deliver the most alpha, the lowest volatility, or the best return. Using artificial intelligence is one way for innovative asset managers to get an edge on the competition, but they must ensure that the solution they choose is finance specific and not vulnerable to regime shifts. Boosted.ai has focused exclusively on creating predictive machine learning algorithms for capital markets.
Interested to hear more? Reach out to us here