Executive Summary:
- Artificial intelligence portfolio optimizations can help institutional investors with volatility and turnover portfolio needs
- Significant reductions in volatility and turnover can come at only minor reductions to return (and sometimes improvements in other metrics like Sharpe and alpha)
- Our optimizers led to a 576 bps drop in volatility, a 92.26 percentage point drop in turnover, with only a 118 bps drop in alpha
- Turning on all our machine learning optimizers can send turnover under 100%
Machine Learning In Investment Management
Machine learning is a powerful tool for investment managers to add performance to their portfolios. We’ve previously discussed methods like factor timing, portfolio construction, and relative valuation as ways a portfolio manager can improve their results. Today, we will highlight how PMs can utilize portfolio optimization to achieve their investment goals.
All Boosted.ai artificial intelligence models start with an asset manager selecting the variables they think are important in stock picking. These can be incredibly varied, incorporating alternative data like credit card data or store visits, traditional balance sheet variables, options data, their own equity data, Federal Reserve data, and more. Our machine learning takes all these disparate sources of information, runs millions of comparisons on them, and produces a model that dynamically ranks stocks into 5-star quantiles or buckets. The stocks that our machine learning predicts will do well are in Q1 and the stocks that it predicts will do badly are in Q5.
Applying AI Portfolio Optimizations: Reducing Risk and Turnover
The core of our Boosted Insights models compares stocks in Q1 against stocks in Q5. If the quantile spread (the difference in Q1 – Q5) is high, the PM can be confident they have created a model that should perform well. We generally define a good model as one with quantile spread of above 10% – that is, if you subtract the performance of Q1 from the performance of Q5 and have a score above 10%, the core of the model is good.
However, a model with good quantile spread might still not meet an asset manager’s goals. Below, we will show how a PM can use artificial intelligence portfolio optimization to hit their investment goals. The PM in this case is a long-only manager that seeks to keep their risk and turnover lower.
In the S&P 500 sample model below, the quantile spread is 16.05% (24.04% – 7.99%). The alpha is 6.45%, return is 24.99% (against the SPY benchmark at 17.26%) and the Sharpe is 1.02. This model was created using our proprietary machine learning algorithm, Battle Royale Noise Reduction. It rebalances quarterly, retrains quarterly and has a 21 day investment horizon. The PM likes this portfolio, but thinks that the volatility of 24.07% is a bit high.
Portfolio 1: Reduce Risk
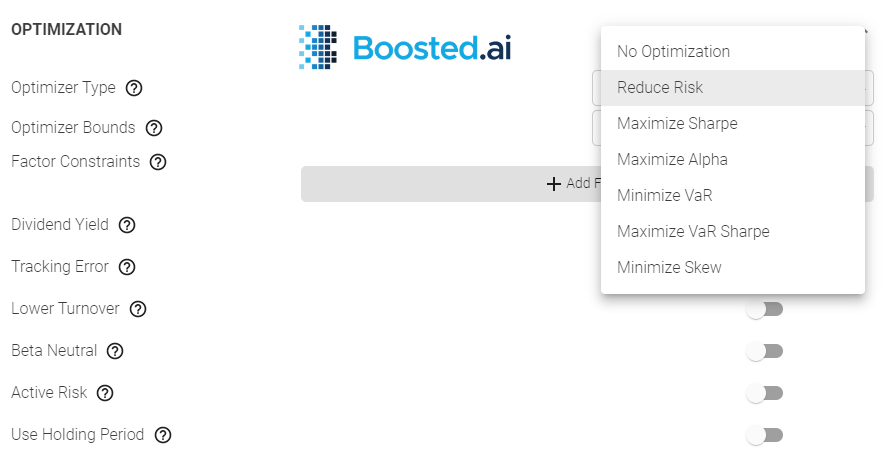
In our first portfolio optimization, Reduce Risk Portfolio, we use our AI portfolio optimizers to add one of our many optimization types: Reduce Risk.
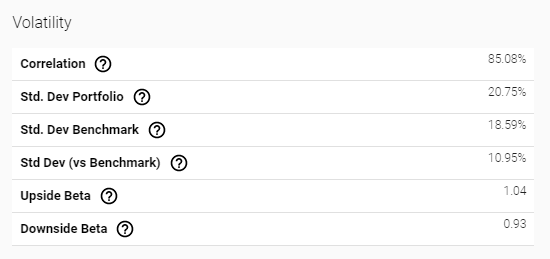
In making this change, we see the volatility drop to 20.75%, a reduction of 332 bps. The alpha and return drop in this portfolio as a result of the reduced risk, to 4.92% alpha and 21.34% return (which still beats the benchmark by 408 bps), but the manager is comfortable with these changes for the reduction in volatility.
However, the portfolio manager is also seeking to reduce turnover and this portfolio, with 318.22% turnover, is actually slightly higher than the original model’s 307.28%. We can fix that!
Portfolio 2: Reduce Risk + Turnover Optimization
Our next portfolio optimization, Reduce Risk + Turnover Optimization, takes the above model (with the Reduce Risk optimizer added) and adds a turnover optimizer as well.
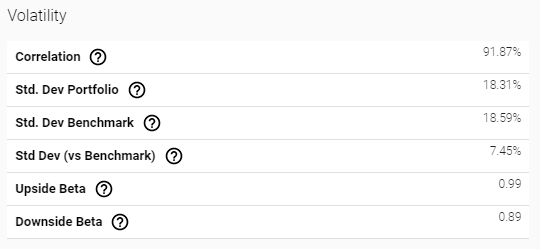
This portfolio seeks to reduce turnover by marking it as important to the end user. Sitting at the middle of the range (low meaning that turnover is not of great importance to the asset manager, and high meaning that turnover is of great importance to them), turnover will be reduced more than if this optimizer were not turned on. It improves on Portfolio 1 in some return metrics as well – this portfolio has alpha of 5.27% and Sharpe of 1.12. Its return is 20.94%, but again, the portfolio manager is comfortable with this slight drop for the continued drop in volatility. This portfolio has 18.31% volatility (576 bps down from the original portfolio) and its turnover is only 215.02%.
Portfolio 3: Reduce Risk + Minimize Turnover
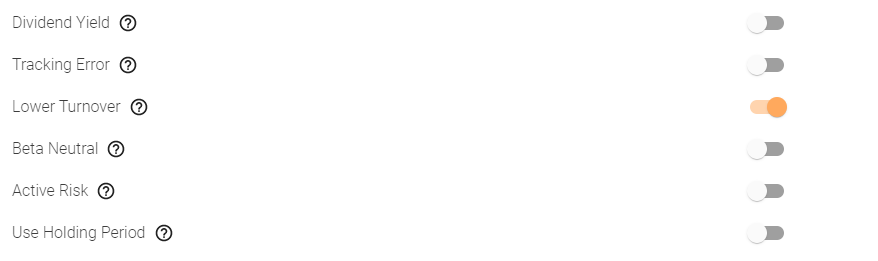
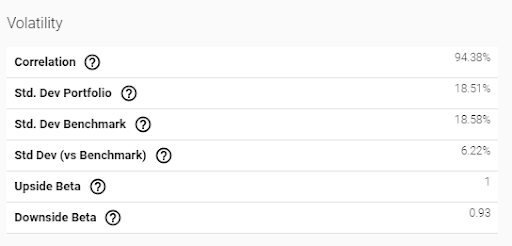
It’s possible to take turnover significantly lower. In this final portfolio, Reduce Risk + Minimize Turnover, the PM managed to utilize our suite of AI optimizers to squeeze turnover down to 97.74%. In this portfolio, the manager keeps the same constraints as the Reduce Risk + Turnover Optimization one before and adds additional optimizers. Turnover Buffering is one technique to reduce the turnover, but the PM also adds a Lower Turnover setting, and ups the Turnover Importance from the previous model to high.
This all translates to a model with only 97.74% turnover while volatility rises slightly to 18.51% (it should be noted that the volatility versus the benchmark drops to 6.22%). Of course, with fewer trade opportunities, alpha and return do drop, here to 2.36% and 18.38% respectively. These tradeoffs are decisions all portfolio managers have to make individually.
Takeaways
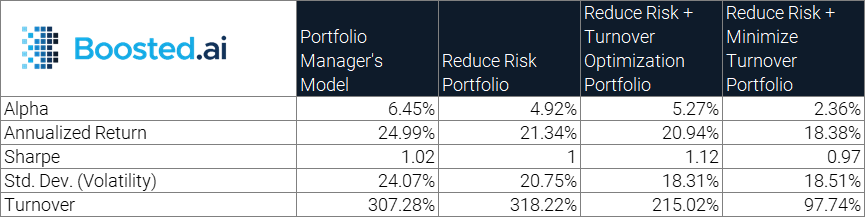
The above table showcases our results. It shows that AI optimizations can help portfolio managers achieve their specific goals around volatility and turnover. There are pros and cons to all methods, but implementing AI in their investment process can help managers see and account for their specific portfolio goals. This is just one method of using our machine learning optimizations to improve portfolio outcomes. We have dozens of different settings to experiment with and backtest until the portfolio manager is comfortable using Boosted Insights to trade live money.
If you’re curious and want to dive under the hood of how we achieved these results, reach out today. We are happy to construct a bespoke model that meets your specific portfolio needs.