Regime Shifts and Quant Portfolios
Machine learning can be useful for a lot of things – picking out movies to suggest, performing live translation, even (one day) fully self-driving cars. In investment and finance, the application of machine learning is still relatively new, but it offers exciting results, especially in the age of uncertainty we find ourselves in, here in 2020. We have previously discussed how using machine learning helped models pick up on Coronavirus risk factors. Today, with the United States election behind us and announcement of an effective Coronavirus vaccine from multiple pharmaceutical companies, we’d like to highlight how our machine learning portfolio construction methods can adapt to regime shifts.
After the election, Pfizer (PFE) unveiled a Coronavirus vaccine purported to have 90% efficacy. The announcement of a possible vaccine shifted the regime in the largest one-day reversal of momentum to value since the financial crisis in 2008. John Quigley of Great Lakes Advisors told Bloomberg about this momentum crash: “Events happened that statistically never could happen.”
This shift rattled quant funds, some of which were already experiencing issues. Two of the most well known quant hedge funds, Renaissance Technologies and Two Sigma Advisers, both saw losses that capped off a wild October. RenTec’s long-biased fund, Bloomberg reported, was down 20% through October. The same report said that Two Sigma lost 11.5% in 2020 through October. Considering these quant blowups, our Boosted.ai machine learning models had some intriguing results.
Boosted.ai’s Results
We can illustrate how Boosted.ai’s machine learning adapts to regime shifts, by examining one of our sample models, which is benchmarked to IWB (a Russell 1000 ETF).
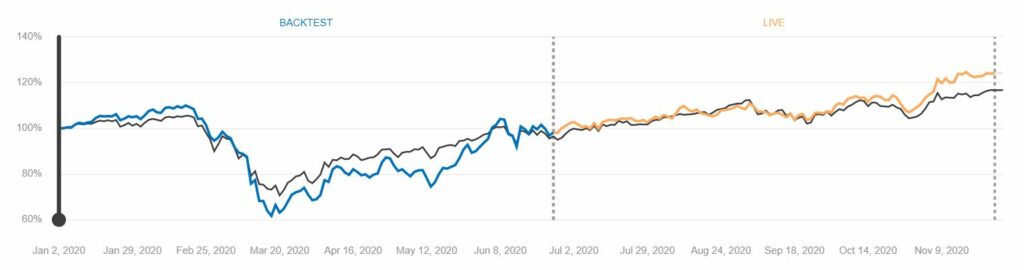
It has 100 longs and shorts, is 100% net long, and rebalances on Mondays at the open. The core of all Boosted portfolios is a two-step process. The first step is ranking all the securities from 0 to 5 stars, and then using the rankings to construct a portfolio that matches the users investment and risk constraints. This Russell 1K model performed well in election week, with longs outperforming shorts 6.21% vs 0.99% (on average), but ultimately slightly trailed the benchmark as of the close on Friday November 6. Given how “up” the market was – the result was in line with expectations.
Things got more interesting over the weekend – which is when the largest one-day reversal of momentum to growth occurred. The picks (from the previous Monday) were exposed to that momentum to growth reversal – but the portfolio construction and machine learning optimization mitigated the damage. The ML factor constraint process (described here) weighted the securities such that the portfolio was up 5.75% that weekend (Friday close to Monday open) versus the IWB being up 2.33%.
After it rebalanced on Monday, November 9th, the model outperformed IWB again through to the open on November 16, up 1.91% vs IWB -0.25%.
Takeaways
So you had three different market environments in two weeks, the week of November 2nd to November 6th, which saw momentum outperformance – where the stock picks made by the machine allowed the model to almost match an aggressively up market. Then you have the momentum to value rotation, where the machine learning factor optimizations and constraints allowed that same portfolio to be weighted such that it massively outperformed (5.75% vs 2.33%). And finally, you have the following week where some of that momentum to growth rotation was undone – and the model still outperformed. The ability to not only recognize but adapt to quickly changing conditions is the reason why machine learning can be useful for a lot of things – including investing in unpredictable environments.